13795
How Google Query Refinement Is Changing SEO

Your Keywords Are Not What You Think They Are
How Google transforms search queries and what it means for SEO

By: Aleh Barysevich
February 28th, 2017
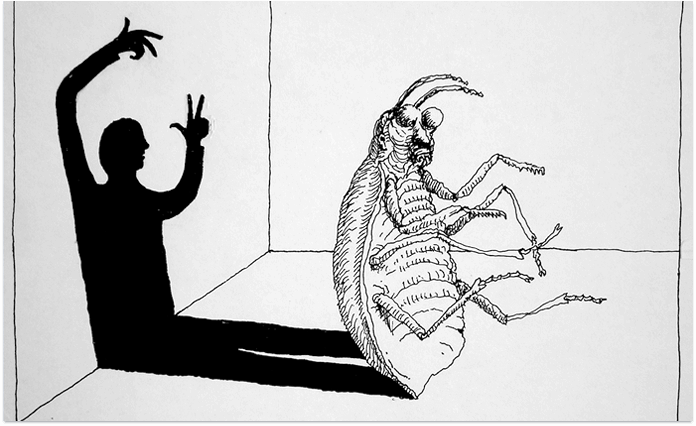
A 1915 illustration to Franz Kafka's "The Metamorphosis"
In Franz Kafka's novella "The Metamorphosis", a man wakes up one morning to find out he has turned into a giant insect. If keywords were existentialists, they could be experiencing something similar as, having been typed into Google's search bar (or any other search engine), they go through numerous transformations to become new, modified versions of themselves — the queries that Google thinks are a better representation of the searcher's intent.
To illustrate what I'm saying, let me ask you for a tiny favor. Go on and type in "oscar" into Google search. No, seriously, do it. I'll hang in here while you're at it.
Chances are all of the top results you got are about the 89th Academy Awards that took place a few days ago. Some of the results likely do not mention the word "oscar" (the only word you typed in, remember?) at all. Somehow, Google search knows you're looking for the specific Oscars award rather than general information about the ceremony, Oscar the name, or Oscar anything else — and transforms your query behind the scenes into something that barely resembles the original.
In this post, I'll look at how this process may work, and what it implies for SEOs.
Associations, relevance, and search volume
Google's recent patent on providing search query refinements offers lots of insight on how Google may deal with search queries that are ambiguous, too general, too narrow, or lack context (like the "oscar" search you just did). The patent describes the system for transforming the search queries into a better-phrased version of themselves, so that Google can provide exactly the search results the user wants.
According to the patent, when Google receives search queries, it will go and grab web documents from its index that it associates with the query, based on the keyword the searcher typed in. It will then look at the pages it extracted and the concepts, or semantic clusters, they are associated with. If it finds out that the results belong to a few very different concepts, it will likely conclude that the search queries that re ambiguous and would benefit from a refinement.
Let's try and see how the actual refinement process works on some queries, with "oscar" again being our example. When you type in "oscar" in Google (or another search engine such as Bing), it will go on and collect a number of search results that literally match the query. For the purpose of this example, let's say this number is 100. Each of the 100 search results is assigned a score estimating their relevance to the original query ("oscar"), likely based on the traditional on- and off-page SEO factors. Next, Google (or the other search engine) will dig deep into the search term to identify the topics, or semantic clusters, these pages belong to; for "oscar", such clusters may be: "Academy Awards", "Oscar the name", "oscar the fish", and perhaps a number of smaller ones. Somehow, Google has to figure out which of these concepts you are interested in — and this is where things get interesting.
According to the patent, Google will next turn to its "association database" — the place where it stores past queries, web pages, and the associations between the two. To each association, Google assigns a weight — the degree of relevance of the page to the query, multiplied by the query's frequency, or search volume. Next, Google may look up the top results it just extracted for your "oscar" query in this database. It will look at the past queries associated with each of the results, and the weights of these associations. The highest scoring associations from the highest scoring clusters will be picked as candidates for the refined query.
Remembering that weight is relevance multiplied by search volume, you are probably starting to understand why, at this particular moment, your search for "oscar" turned into something like "Academy Awards ceremony 2017".
It is worth noting that Google will often pick more than one cluster, or topic, to refine the query. This can be especially obvious when the possible clusters associated with the original keyword have equal, or close to equal, weight — that's when Google will let you decide which of the topics you're interested in.
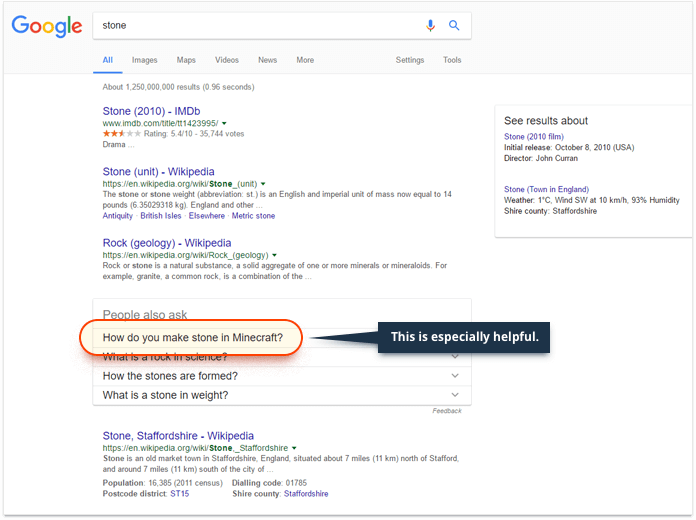
Context
Another patent sheds light on the role of context in refining a user's queries. Context, in this case, is a collection of words and phrases, associated with a single domain. The data for such "contexts" is obtained from large corpuses of training materials, and then likely improved and expanded with machine learning. These contexts help Google both index information and serve searchers better. For the former, Google divides "the universe of communications" into domains, which are similar to the clusters discussed above. By looking at a web page and the words and expressions used within it, Google can then easily figure out which context the page can be attributed to, based on the intersection of the words in a certain context and the content in the page. So when it looks at a page that contains terms like "the Academy", "Academy Awards", and "best motion picture", it will go "aha, this page's context is Oscars the award".
On the user end, to determine the context of their query, Google will look at things like the user's immediate past searches, voice search, and if necessary, their entire search history. In other words, if your search history implies that you are particularly interested in the oscar fish, Google may give the fish-related cluster a boost when you search for oscar, based on the contextual information it has about you as a searcher. So in fact, your search for "oscar" turns into "please search for "oscar" bearing in mind all you know about me, Google".
Context 2.0
Your search and browser history are not the only kind of "context" Google might use to refine your queries. Several recent patents imply that more intricate details about the searcher, such as movies they saw or music they listened to may be used to refine queries and the results returned. Real-time context, like the movie that's currently on, may also be used when the user makes a voice query.

For example, a user can input a natural language query at a device, such as the spoken query, "When have I seen this actor before?" while viewing particular content, such as the movie "The Social Network".
According to these patents, Google may also monitor what's on TV in your area, and look for queries that might be related to that information. So, if you search for "social network" and the movie "The Social Network" is currently on in your area, that may influence the search results you receive. For example, that may give preference to the "Social Network the movie" cluster over other semantic clusters associated with your query.
Location
Location, of course, is a kind of context, but it deserves a place of its own on this list. Location already affects a massive part of queries, and it is particularly important for businesses who are taking advantage of paid search in order to get their websites to rank locally. But Google's use of location to refine queries may soon grow way beyond its current state.
You probably already know that if you search for "Starbucks", "Walmart", or a certain entity that may imply that you’re interested in a physical location of a business, Google will show you the local pack and adjust the organic search results to help you find the physical place you're (likely) looking for. This can be taken a step further as Google may look at query patterns and associate them with entities that are close to the location of the searcher. So, if you asked Google "what time does Starbucks open?", or even, "what's this park called?", while the entity you are referring to is unambiguously close, Google will be smart enough to give you the answer.
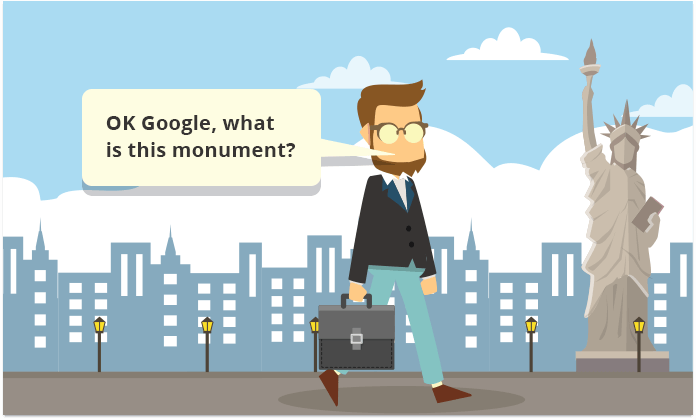
So next time you pass by a restaurant — let's say it's called Zio Pepe — wondering if it's any good, try asking Google "any ratings for this place?" This query may be turned into something like "zio pepe ratings", and you won't have to worry about how "zio" is really pronounced in Italian.

For example, a user that does not speak German can be on vacation in Zurich, Switzerland and can submit the query [opening hours], while standing near a restaurant called "Zeughauskeller," which may be difficult to pronounce and/or spell for the user. As another example, implementations of the present disclosure enable users to more conveniently and naturally interact with a search system (e.g., submitting the query [show me lunch specials] instead of the query [Fino Ristorante & Bar lunch specials].
Query substitution
Another recent patent filed by Google focuses on substitution terms and synonyms for query refinement. The process includes identifying a concept in the user's query and figuring out if the same concept can be phrased differently (without distorting the meaning of the query) to provide better suited search results.
To see how this works, let's do a Google search for "UK President". If Google was simply looking for the two words mentioned in the query, "UK" and "President", the results could include articles about presidents of other countries visiting the UK. Instead, Google identifies that the searcher is likely looking for a different kind of information, and the word "President" is actually a mistake the searcher made when they really meant "Prime Minister". In the given context, "Prime Minister" is synonymous with "President"; combined with "UK", it also represents a known entity. All of that makes it a good substitution term.
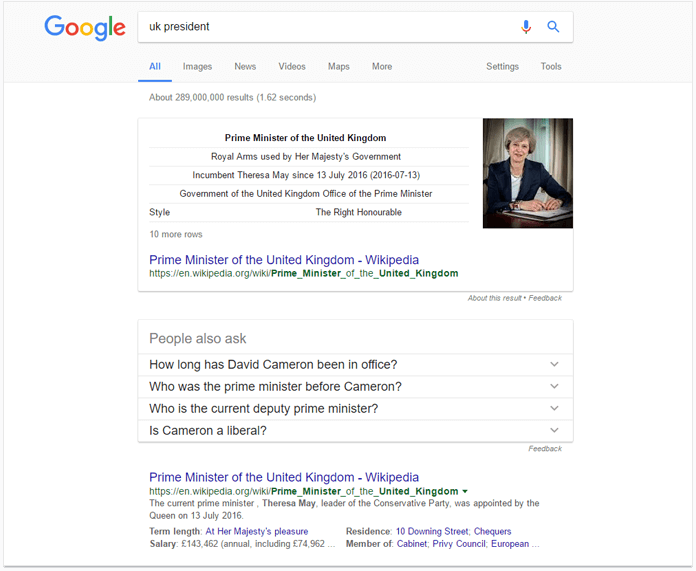
(Note how Google has bolded "Prime Minister" in the URLs in the SERP, and how the term "President" appears nowhere among the top results).
Word2vec
There's been a lot of discussion of word2vec in the SEO space lately, sparked by the opinion expressed by numerous SEOs that it may be the technology used by Google's RankBrain. And this opinion has its grounds: many of the folks working on RankBrain also worked on word2vec, and many of the descriptions of the two projects are nearly identical.

The word2vec tool takes a text corpus as input and produces the word vectors as output. It first constructs a vocabulary from the training text data and then learns vector representation of words. The resulting word vector file can be used as features in many natural language processing and machine learning applications.
Before we get down to the ins and outs of word2vec, let's have one thing settled. The SEO folk has been talking about term vectors and the vector space a lot lately. While these concepts may be complicated in theory and sound like you need to take a calculus course to grasp them (and you sort of do if you want to get to the bottom of things), their individual visualizations are in fact surprisingly understandable.
Let's look at a simple example. Say you've got a set of terms that you need to split in two clusters, "vegetables" and "meat". Not all of the words name a vegetable or a kind of meat, but you have to do this anyway, grouping words that occur in the meat context more frequently (such as "smoked") into the "meat" cluster, and vice versa.
Here's what a visualization of these word vectors may look like.
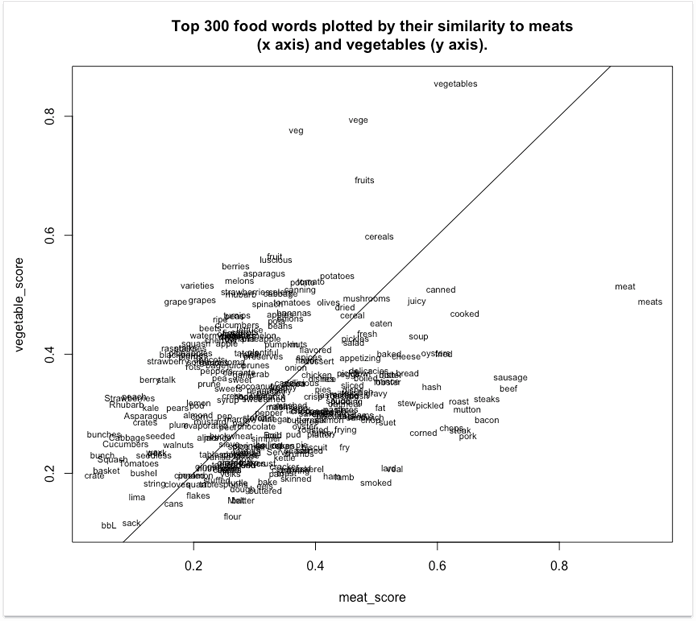
Source: http://bookworm.benschmidt.org/
As you see, the terms with high "vegetable" scores are towards the top, and the ones with high "meat" scores towards the right. Close to the line in the middle are the more neutral terms — ones that occur in both contexts equally often.
It is important to note that clustering terms into semantic groups is far from the only thing word2vec can do. Another important aspect is identifying relationships between terms by calculating the physical distance between their vectors. Above, you can see that "meat" and "meats" appear next to each other, and are therefore close in meaning. "Chops", "steak", and "pork" are bundled even closer together. Note that the words that are next to each other don't have to be synonyms. They can be terms that frequently appear close to each other, like "banana" and "apple".
Now, let's take this another step forward. We've figured out that we can subtract the vectors from each other to identify their relatedness (the smaller the distance, the more related they are). But what if we add two vectors together? And then subtract another vector from the sum?
Apparently, this is exactly what Google does for some queries.
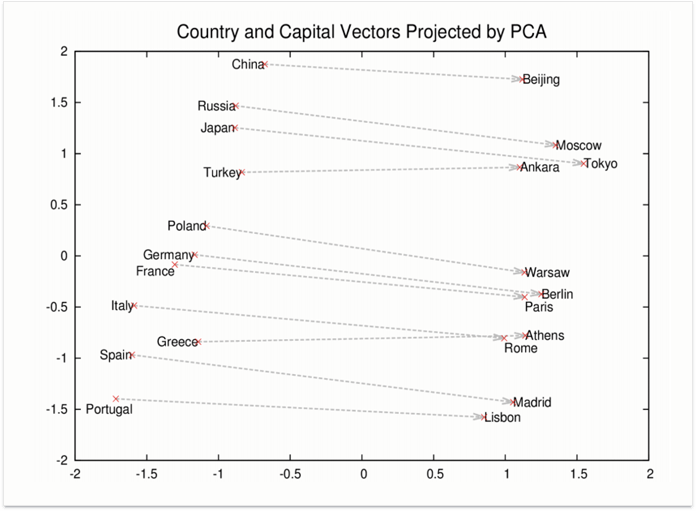
For the vectors above, the equation Rome - Italy + China would equal Beijing. This is, in fact, vector talk for the question "What is the entity that has the same relationship to China as Rome to Italy?", or, simply, "What is the capital of China?"
Here's an example of how word2vec is being used in query processing. Assume for a moment you forgot the word for "toe" (and you desperately need that word). Thanks to term vectors, you can go on and google "feet fingers", and however weird that sounds, Google will get what you mean. It will figure out that fingers are part of the "hand" concept, and modify the query into something like "What has the same relationship to feet as fingers to hands?" (or, in vector talk, "foot + finger - hand") and will look for the missing piece in this association. Thanks to that, you will see exactly what you're looking for (instead of a list of pages that mention both "feet" and "fingers").
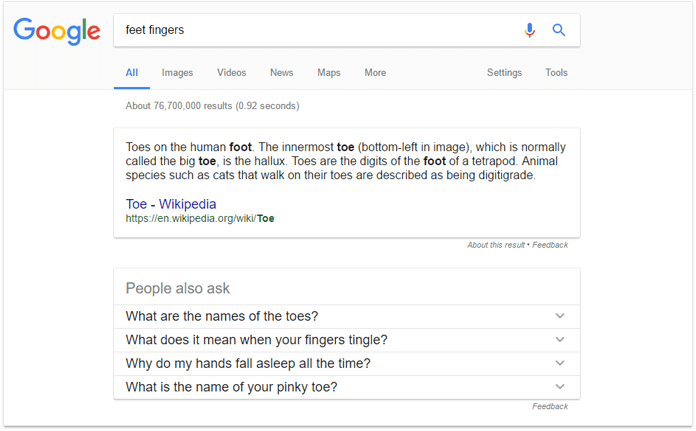
See how they bolded "toe" in the featured answer without a single mention of the word "finger"? Smart, huh?
Entities
Entities are elements of Google's Knowledge Graph — specific objects that Google knows some facts about, such as persons, places, and things. A business, a celebrity, or a plant can be entities. The great thing about entities is that Google knows quite a lot about them, which lets searchers instantly find an entity by a certain fact.
Thus, a search for something that Google considers to be a unique property of a certain entity will produce search results about that entity. This way, "biggest city in the world" may be transformed into "Which entity has the unique property of being the biggest city in the world?" and match the entity of Tokyo. Similarly, “Google CEO” will match Sundar Pichai.
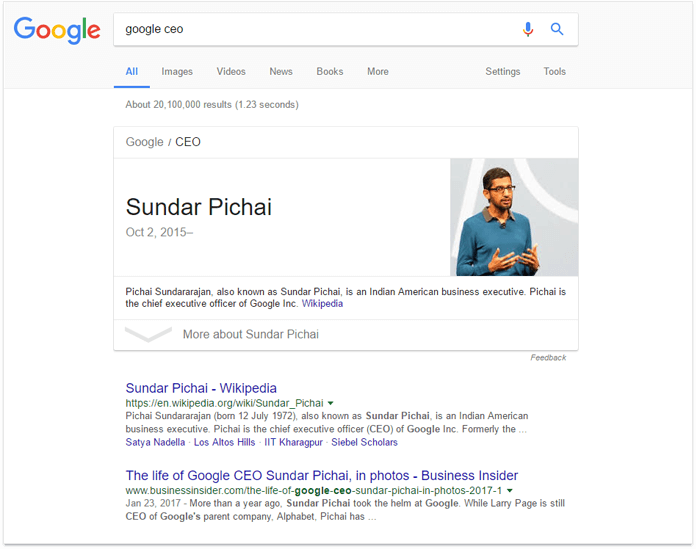
Kind of makes you want to have a unique, objective characteristic for your business' entity, doesn't it?
Interestingly, user behavior signals may impact such entity-based search results just like any other kind of results. Say, if Google finds two entities with similar weight that match the query, it will typically display results about both in the SERP. If you search for "Joe's NYC", provided that you've never searched for it before, you'll get results about a number of businesses with the name: Joe's bar, Joe's pub, Joe's coffee shop, and Joe's pizza place. But once you click on a certain result — say, the pizza place — Google may store this entity as your preferred one in the context of this search. So when you run a similar search again, that preferred entity of yours will likely appear at the top of the results, and other entities may be eliminated from the SERP altogether.
Final words
The above suggests that Google is rapidly getting cleverer (even human-like, if you will) at figuring out the meaning behind keywords, rephrasing those keywords, and producing better search results in response. We may see this as bad news or as good news, but there's little we can do but adapt.
One concept I want you to take away from this article is that the search results users see are not just a combination of the query and the ranking factors. In-between, Google may modify the query so that it can answer it better, and this modification process may be very different for various queries — and even for the same query made at different points in time (imagine searching for "oscar" a few months from now).
So is there anything SEOs can still do now that we're tangled in this semantic web? Sure. Here are a few tips.
1) Get on the Knowledge Graph. While there's no magical formula that will get you there for sure, there's a number of steps to take that will significantly boost your chances of winning a Knowledge Graph listing for your business. Here are the steps for the local Knowledge Graph panel, and the ones for the more universal Brand Knowledge Graph.
2) Keep fighting for those rankings. I've always been surprised by how some SEOs begin to doubt the value of rankings as an SEO KPI because of the growing personalization of search. Don't forget that when someone runs a search for your target keyword for the first time, you've got to do your best to appear among the top results. If you do, and if the searcher clicks on your listing, you're becoming their preferred entity, and their subsequent searches will likely include your business as the top, if not the only, entity in the results. Otherwise, if your competitor ranks at the top and the searcher clicks on their listing, you probably lost that client for good.
3) Monitor your niche closely. It's pretty clear now that Google may use different ranking factors (and different query refinements) for different niches and even individual queries. The universal approach to SEO with its foundation on links and site structure still has a say; but in the context of a certain query, it might be outweighed by other factors, that are specific to that query or niche.
This is where SERP intelligence comes in, and with SEO PowerSuite's Rank Tracker, for example, we’ll see complete SERP History — an archive for the top 30 search results for every ranking check you run. If you look at the SERP fluctuation graph, you'll be able to instantly spot important changes in the SERPs for your every keyword during each of your ranking checks. The red spikes on the graph will instantly let you know there's been an important change in the SERP that you need to look into. This may indicate that Google's started to interpret the query differently, or that it's begun to look at different ranking factors. In either case, it's a good idea to check with the SERP and see what's changed — so you can be the first to adapt.
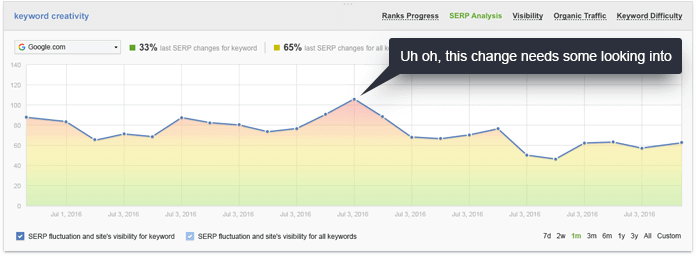
With just about as many tools as rum on Blackbeard’s fleet, I cannot recommend SEO PowerSuite enough for all things keyword research for SEO. The Rank Tracker tool analyzes the websites of your top 10 ranking competitors for topic-relevant terms with the TF-IDF algorithm, which will help you build up your authority in semantic search results.
Ready with the killer keyword list? Download Rank Tracker and test out the array of available tools, or better yet, grab yourself a license to unlock a real arsenal of options that’ll see your website rank like never before.
Now, over to you. What are your thoughts on the impact of semantic search on SEO and predictions on its future? I'm looking forward to your opinions, ideas, and questions in the comments below.